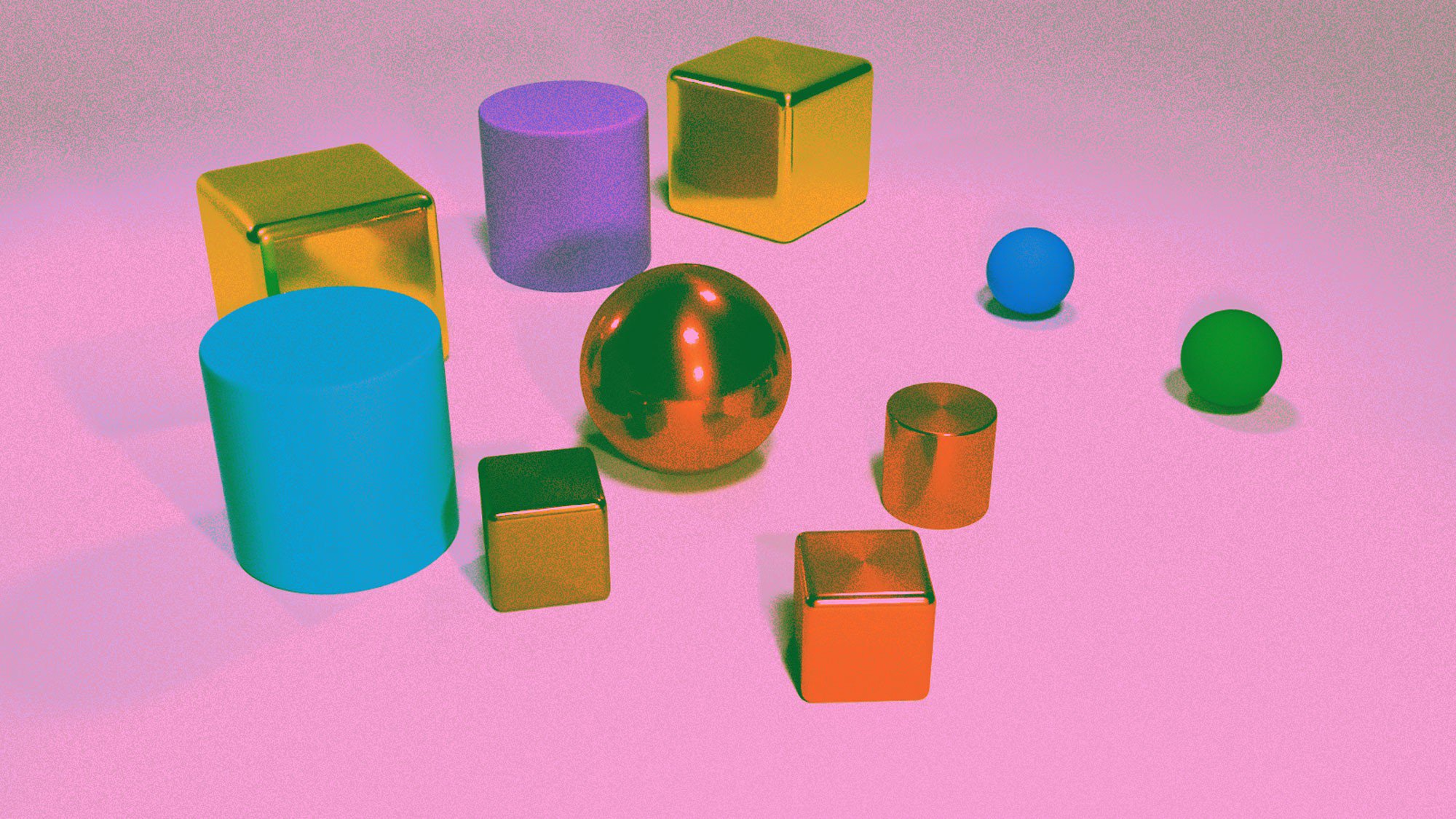
09 Apr Neuro-Symbolic Learning Frameworks: Can the machine reason like a child?
It was a late night in the office, but I was still excited to read this paper out of MIT Professor Josh Tenenbaum’s group – it highlights an approach that somewhat bridges the divide between “symbolists” (develop intelligence the way a child learns) and “connectionists” (using patterns from data to become intelligent). Not only are the approach and its results encouraging, but I am energized that divergent schools of thoughts now have the intellectual space and will to collaborate. Josh is a collaborative researcher.
I’ve personally been more interested in the symbolists’ approach for some time as I believe, having been raised on Minskian fare, that it’s the only real approach to artificial intelligence. It’s more intellectually pure – the endgame ultimately is that we want to code our machines to efficiently reason the way we do. There will inherently always be limits to what a highly supervised learning approach like deep learning can achieve given that it requires large amounts of data. By contrast, a child learns by using small amounts of data.
In recent years, however, key breakthroughs in deep learning have enabled the neural network approach to become quite useful in several applications, ones that many of our portfolio companies have been using (i.e. computer vision). It is a more practical approach, for now.
I’m hopeful that further research along the lines of this neuro-symbolic approach will yield more practical applications of intelligence that can reason and not just see or analyze. Cognition is the path to true artificial intelligence and the applications of machines being able to truly reason based on highly limited data sets will be transformational. I can’t wait to help build companies using some of these future techniques.